Serious pregnancy complications often show their first symptoms midway through pregnancy. High blood pressure and dark urine around 20 weeks can indicate preeclampsia, while excessive thirst and frequent urination at 24 weeks may signal gestational diabetes. Though blood tests can confirm these conditions, that typically happens only after symptoms appear—when “the damage has already been done,” says Surendra Sharma, an obstetrician and molecular biologist at the University of Texas Medical Branch.
To intervene earlier, researchers are working on a tool to detect complications before symptoms begin. A promising new approach comes from Carlos Palma and his team at the University of Queensland. Their work focuses on placental extracellular vesicles (EVs)—tiny pockets of molecules released by cells throughout the body that reflect their state and activity.
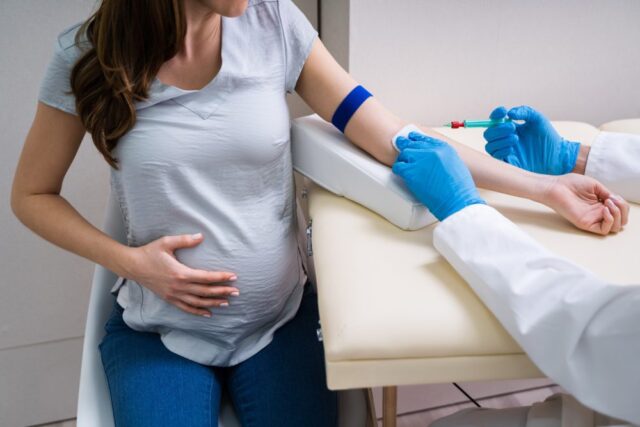
A lot is still unknown about the precise relationship between health and EVs for various tissues and cell types, but researchers have linked disease and distress to changes in both the composition and the quantify of EVs released by the placenta. Focusing on the latter, Palma’s team developed a sensor to measure the concentration of placental EVs in maternal blood, aiming to predict risks well before symptoms show.
The team analyzed blood plasma from 201 women around 13 weeks into their pregnancies, on average. Half of the women had normal pregnancies, and the other half developed a serious complication. These included preeclampsia, gestational diabetes, preterm birth, and others. Using machine learning, they identified two key protein markers—placental alkaline phosphatase (PLAP) and CD9—as the best indicators of risk.
They then designed a sensor using magnetic beads coated with PLAP antibodies. These beads, engineered with enzyme-like properties, react with a color-changing dye (TMB), turning it blue in the presence of target EVs. When applied to a CD9-coated surface and washed, only EVs expressing both markers remained. The deeper the blue, the more EVs were present, indicating a higher likelihood of complications.
This method proved particularly effective in identifying women at risk for gestational diabetes. Andy Powell, a biochemist at Liverpool John Moores University, praised the approach, comparing it to a pregnancy test—simple but powerful. He also noted the advantage of using stable artificial enzymes over biological ones.
However, some experts remain cautious. While the test measures EV quantity, it doesn’t identify the specific messages those vesicles carry. “They haven’t really looked in detail at what signals these EVs might be carrying,” said Iain Dykes, Powell’s colleague.
Sharma also expressed skepticism about relying solely on EV counts, which have shown inconsistent results across studies. He believes the technology could be stronger if tailored with disease-specific markers: “It’s a very wonderful starting point,” he said. “We need to go to the finish line.”